Markdown Rstudio
Interactive documents are a new way to build Shiny apps. An interactive document is an R Markdown file that contains Shiny widgets and outputs. You write the report in markdown, and then launch it as an app with the click of a button.
RStudio Support. New articles New articles and comments. Including verbatim R code chunks inside R Markdown. Open a new.Rmd file at File New File R Markdown. Use the wizard that opens to pre-populate the file with a template 1 Write document 2 by editing template Spell Check Publish Show outline Knit document to create report 3 Use knit button or render to knit Examine build log 6 in R Markdown console Preview Output 4 in IDE window. I outline how I structure analytical project folder systems and some hints for matching R Markdown documents to a corporate style guide including adding logos, watermarks, and of course colours. Oct 19, 2016 Mathematics in R Markdown R Pruim October 19, 2016. Math inside RMarkdown. A blog on statistics and R aiming at helping academics and professionals working with data to grasp important concepts in statistics and to apply them in R.
This article will show you how to write an R Markdown report.
The companion article, Introduction to interactive documents, will show you how to turn an R Markdown report into an interactive document with Shiny components.
R Markdown
R Markdown is a file format for making dynamic documents with R. An R Markdown document is written in markdown (an easy-to-write plain text format) and contains chunks of embedded R code, like the document below.
R Markdown files are designed to be used with the rmarkdown
package. rmarkdown
comes installed with the RStudio IDE, but you can acquire your own copy of rmarkdown
from CRAN with the command
R Markdown files are the source code for rich, reproducible documents. You can transform an R Markdown file in two ways.
knit - You can knit the file. The
rmarkdown
package will call theknitr
package.knitr
will run each chunk of R code in the document and append the results of the code to the document next to the code chunk. This workflow saves time and facilitates reproducible reports.Consider how authors typically include graphs (or tables, or numbers) in a report. The author makes the graph, saves it as a file, and then copy and pastes it into the final report. This process relies on manual labor. If the data changes, the author must repeat the entire process to update the graph.
In the R Markdown paradigm, each report contains the code it needs to make its own graphs, tables, numbers, etc. The author can automatically update the report by re-knitting.
convert - You can convert the file. The
rmarkdown
package will use thepandoc
program to transform the file into a new format. For example, you can convert your .Rmd file into an HTML, PDF, or Microsoft Word file. You can even turn the file into an HTML5 or PDF slideshow.rmarkdown
will preserve the text, code results, and formatting contained in your original .Rmd file.Conversion lets you do your original work in markdown, which is very easy to use. You can include R code to knit, and you can share your document in a variety of formats.
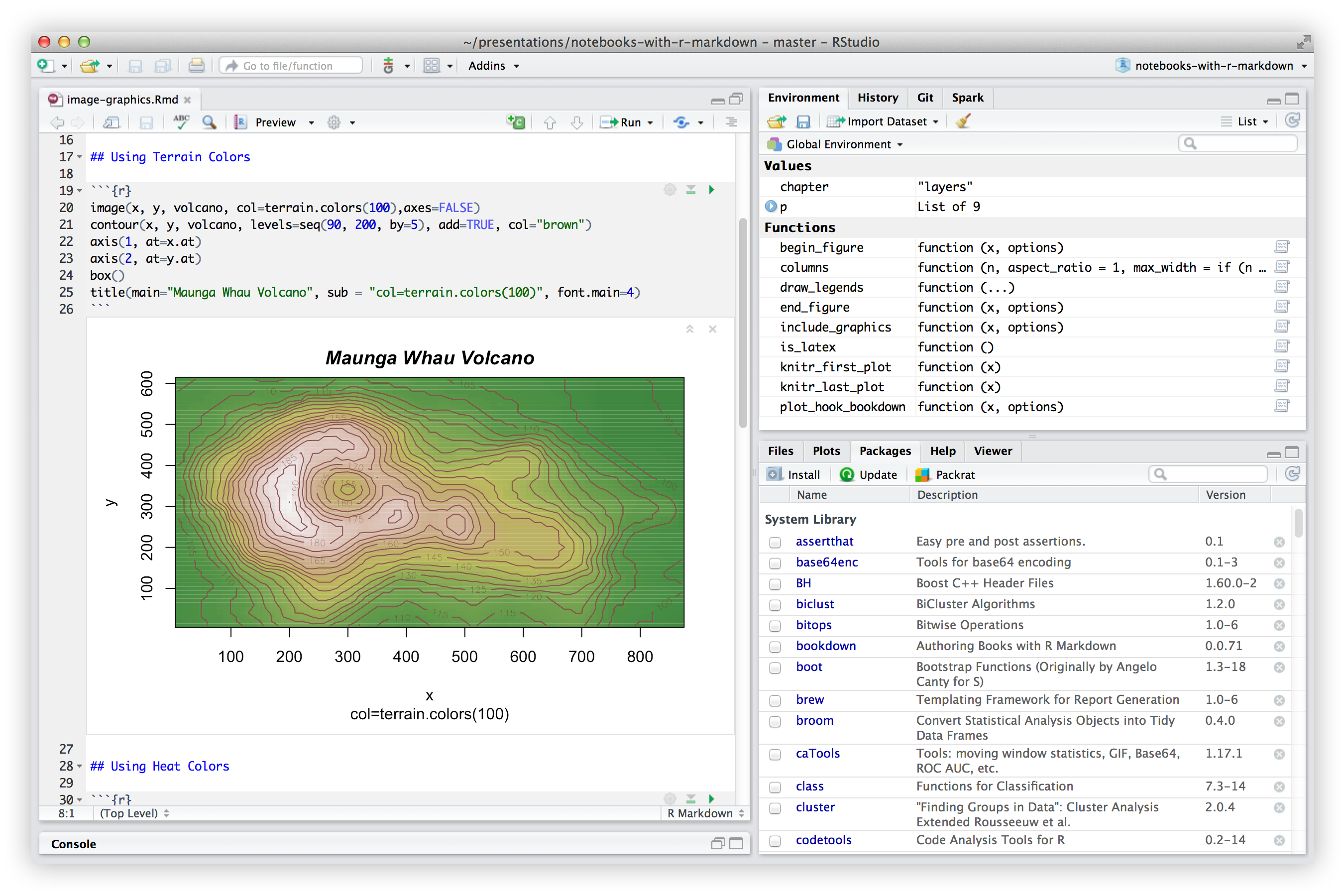
In practice, authors almost always knit and convert their documents at the same time. In this article, I will use the term render to refer to the two step process of knitting and converting an R Markdown file.
You can manually render an R Markdown file with rmarkdown::render()
. This is what the above document looks like when rendered as a HTML file.
In practice, you do not need to call rmarkdown::render()
. You can use a button in the RStudio IDE to render your reprt. R Markdown is heavily integrated into the RStudio IDE.
Getting started
To create an R Markdown report, open a plain text file and save it with the extension .Rmd. You can open a plain text file in your scripts editor by clicking File > New File > Text File in the RStudio toolbar.
Be sure to save the file with the extension .Rmd. The RStudio IDE enables several helpful buttons when you save the file with the .Rmd extension. You can save your file by clicking File > Save in the RStudio toolbar.
R Markdown reports rely on three frameworks
- markdown for formatted text
knitr
for embedded R code- YAML for render parameters
The sections below describe each framework.
Markdown for formatted text
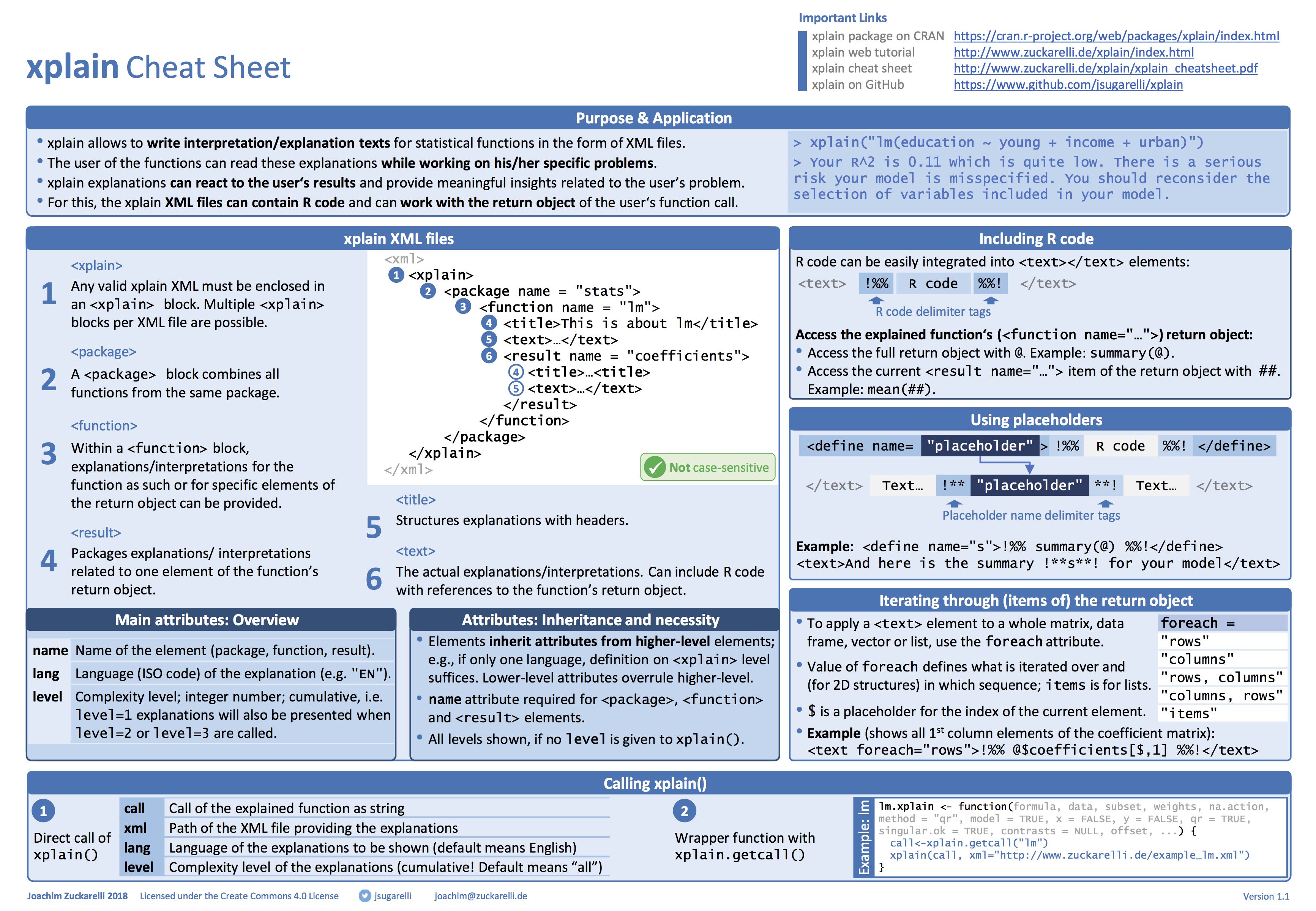
.Rmd files are meant to contain text written in markdown. Markdown is a set of conventions for formatting plain text. You can use markdown to indicate
- bold and italic text
- lists
- headers (e.g., section titles)
- hyperlinks
- and much more
The conventions of markdown are very unobtrusive, which make Markdown files easy to read. The file below uses several of the most useful markdown conventions.
The file demonstrates how to use markdown to indicate:
headers - Place one or more hashtags at the start of a line that will be a header (or sub-header). For example,
# Say Hello to markdown
. A single hashtag creates a first level header. Two hashtags,##
, creates a second level header, and so on.italicized and bold text - Surround italicized text with asterisks, like this
*without realizing it*
. Surround bold text with two asterisks, like this**easy to use**
.lists - Group lines into bullet points that begin with asterisks. Leave a blank line before the first bullet, like this
hyperlinks - Surround links with brackets, and then provide the link target in parentheses, like this
[Github](www.github.com)
.
You can learn about more of markdown’s conventions in the Markdown Quick Reference guide, which comes with the RStudio IDE.
To access the guide, open a .md or .Rmd file in RStudio. Then click the question mark that appears at the top of the scripts pane. Next, select “Markdown Quick Reference”. RStudio will open the Markdown Quick Reference guide in the Help pane.
Rendering
To transform your markdown file into an HTML, PDF, or Word document, click the “Knit” icon that appears above your file in the scripts editor. A drop down menu will let you select the type of output that you want.
When you click the button, rmarkdown
will duplicate your text in the new file format. rmarkdown
will use the formatting instructions that you provided with markdown syntax.
Once the file is rendered, RStudio will show you a preview of the new output and save the output file in your working directory.
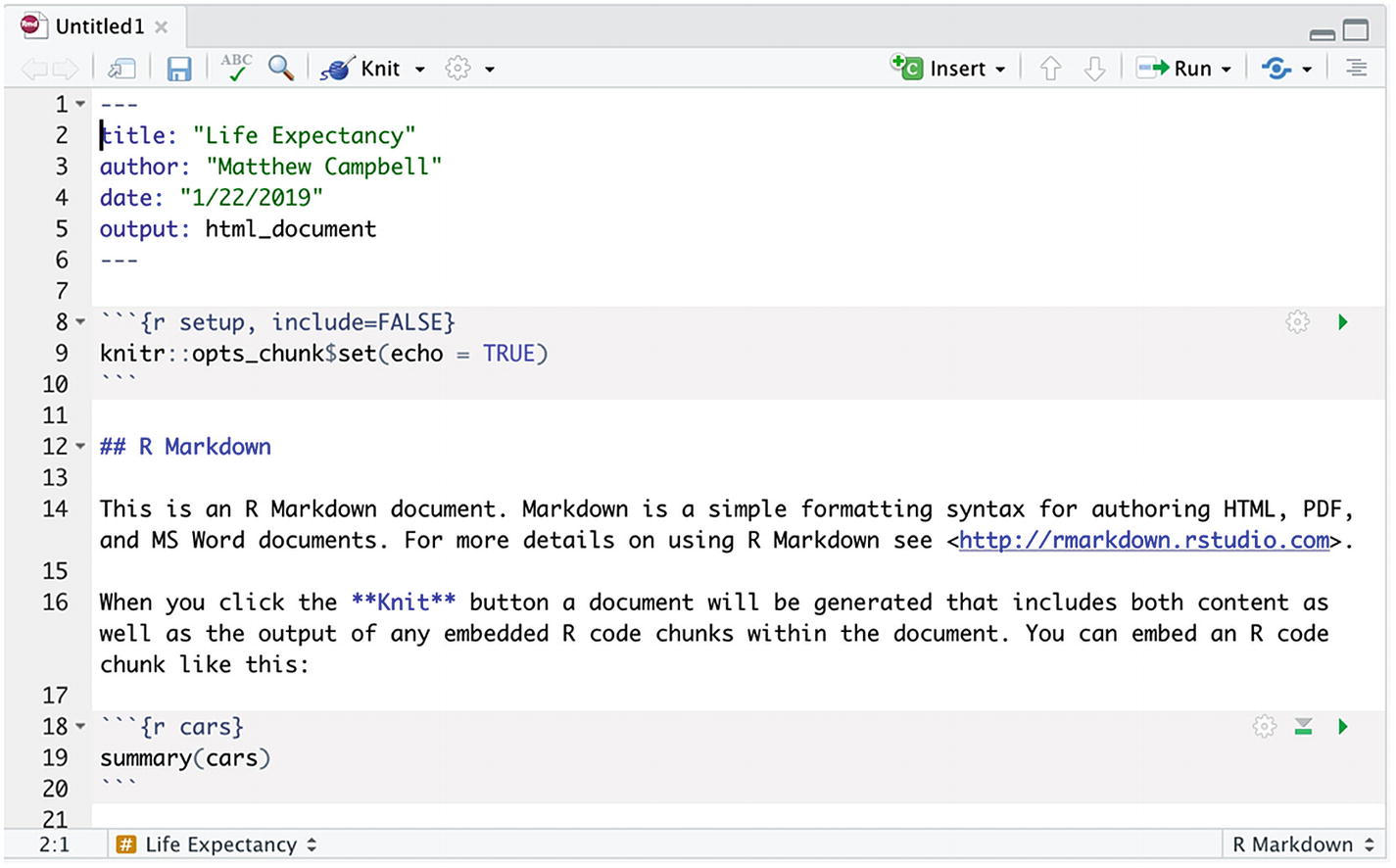
Here is how the markdown script above would look in each output format.
Note: RStudio does not build PDF and Word documents from scratch. You will need to have a distribution of Latex installed on your computer to make PDFs and Microsoft Word (or a similar program) installed to make Word files.
knitr for embedded R code
Markdown Rstudio Example
The knitr
package extends the basic markdown syntax to include chunks of executable R code.
When you render the report, knitr
will run the code and add the results to the output file. You can have the output display just the code, just the results, or both.
To embed a chunk of R code into your report, surround the code with two lines that each contain three backticks. After the first set of backticks, include {r}
, which alerts knitr
that you have included a chunk of R code. The result will look like this
When you render your document, knitr
will run the code and append the results to the code chunk. knitr
will provide formatting and syntax highlighting to both the code and its results (where appropriate).
As a result, the markdown snippet above will look like this when rendered (to HTML).
To omit the results from your final report (and not run the code) add the argument eval = FALSE
inside the brackets and after r
. This will place a copy of your code into the report.
To omit the code from the final report (while including the results) add the argument echo = FALSE
. This will place a copy of the results into your report.
echo = FALSE
is very handy for adding plots to a report, since you usually do not want to see the code that generates the plot.
echo
and eval
are not the only arguments that you can use to customize code chunks. You can learn more about formatting the output of code chunks at the rmarkdown and knitr websites.
Inline code
To embed R code in a line of text, surround the code with a pair of backticks and the letter r
, like this.
knitr
will replace the inline code with its result in your final document (inline code is always replaced by its result). The result will appear as if it were part of the original text. For example, the snippet above will appear like this:
YAML for render parameters
You can use a YAML header to control how rmarkdown
renders your .Rmd file. A YAML header is a section of key: value
pairs surrounded by ---
marks, like below
The output:
value determines what type of output to convert the file into when you call rmarkdown::render()
. Note: you do not need to specify output:
if you render your file with the RStudio IDE knit button.
output:
recognizes the following values:
html_document
, which will create HTML output (default)pdf_document
, which will create PDF outputword_document
, which will create Word output
If you use the RStudio IDE knit button to render your file, the selection you make in the gui will override the output:
setting.
Slideshows
You can also use the output:
value to render your document as a slideshow.
output: ioslides_presentation
will create an ioslides (HTML5) slideshowoutput: beamer_presentation
will create a beamer (PDF) slideshow
Note: The knit button in the RStudio IDE will update to show slideshow options when you include one of the above output values and save your .Rmd file.
rmarkdown
will convert your document into a slideshow by starting a new slide at each header or horizontal rule (e.g., ***
).
Visit rmakdown.rstudio.com to learn about more YAML options that control the render process.
Recap
R Markdown documents provide quick, reproducible reporting from R. You write your document in markdown and embed executable R code chunks with the knitr
syntax.
You can update your document at any time by re-knitting the code chunks.
You can then convert your document into several common formats.
R Markdown documents implement Donald’s Knuth’s idea of literate programming and take the manual labor out of writing and maintaining reports. Moreover, they are quick to learn. You already know ecnough about markdown, knitr, and YAML to begin writing your own R Markdown reports.
In the next article, Introduction to interactive documents, you will learn how to add interactive Shiny components to an R Markdown report. This creates a quick workflow for writing light-weight Shiny apps.
To learn more about R Markdown and interactive documents, please visit rmarkdown.rstudio.com.
ModelOps or MLOps (for “model/machine learning operations”) focuses on the real-world processes involved in building, deploying, and maintaining a model within an organization’s data infrastructure. Developing a model that meets your organizations needs and goals is a big accomplishment, but whether that model’s purpose is largely predictive, inferential, or descriptive, the “care and feeding” of your model often doesn’t end when you are done developing it. How is the model going to be deployed? Should you retrain the model on a schedule? Based on changes in model performance? When should you kick off retraining the same kind of model with fresh data versus go back to the drawing board for a full round of model development again? These are the kinds of questions that ModelOps deals with.
Model monitoring is a key component of ModelOps, and is typically used to answer questions about how a model is performing over time, when to retrain a model, or what kinds of observations are not being predicted well. There are a lot of solutions out there to address the need for model monitoring, but the R ecosystem offers options that are code-first, flexible, and already in wide use. When we use this approach to model monitoring, we gain all the benefits of handling our data science logic via reusable, extensible code (as opposed to clicks), as well as the enormous open source community surrounding R Markdown and related tools.
In this post, I’ll walk through one option for this approach.
- Deploy a model as a RESTful API using Plumber
- Create an R Markdown document to regularly assess model performance by:
- Sending the deployed model new observations via httr
- Evaluating how the model performed with these new predictions using model metrics from yardstick
- Versioning the model metrics using the pins package
- Summarize and visualize the results using flexdashboard
- Schedule the R Markdown dashboard to regularly evaluate the model and notify us of the results
Predicting injuries from traffic data
I recently developed a model to predict injuries for traffic crashes in Chicago. The data set covers traffic crashes on city streets within Chicago city limits under the jurisdiction of the Chicago Police Department, and the model predicts the probability of a crash involving an injury.
Markdown Rstudio Tutorial
I work on the tidymodels team developing open source tools for modeling and machine learning, but you can use the R ecosystem for monitoring any kind of model, even one trained in Python. I used Plumber to deploy my model on RStudio Connect, but depending on your own organization’s infrastructure, you might consider deploying a Flask API or another appropriate format.
Monitor model performance
There are new crashes everyday, so I would like to measure how my model performs over time. I built a flexdashboard for model monitoring; this dashboard does not use Shiny but it’s published on RStudio Connect as a scheduled report that re-executes automatically once a week. I get an email in my inbox with the new results every time!
Rstudio Markdown Cheat Sheet
The monitoring dashboard uses httr to call two APIs:
- the city of Chicago’s API for the traffic data to get the latest crashes
- the model API to make predictions on those new crashes
The dashboard also makes use of pins to publish and version model metrics each time the dashboard updates. I am a huge fan of the pins package in the context of ModelOps; you can even use it to publish and version models themselves!
Basic model monitoring should cover at least the model metrics of interest, but in the real world, most data practitioners need to track something specific to their domain or use case. This is why inflexible ModelOps tooling is often frustrating to work with. Using flexible tools like R Markdown, on the other hand, let me build a model monitoring dashboard with a table of crashes that were misclassified (so I can explore them) and an interactive map of where they are around the city of Chicago.
To learn more
Markdown Rstudio
All the code for this demo is available on GitHub, and future posts will address how to use R for other ModelOps endeavors. If you’d like to learn more about how RStudio products like Connect can be used for tasks from serving model APIs to model monitoring and more, set up a meeting with our Customer Success team.
